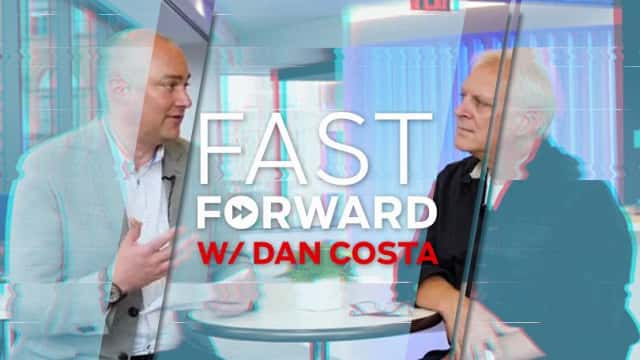
IBM Watson CTO on Why Augmented Intelligence Beats AI
IBM Watson CTO on Why Augmented Intelligence Beats AI https://csuiteold.c-suitenetwork.com/wp-content/uploads/2017/08/ibm-watson-cto-on-why-augmented-intelligence-beats-ai.jpg 640 360 C-Suite Network https://csuiteold.c-suitenetwork.com/wp-content/uploads/2017/08/ibm-watson-cto-on-why-augmented-intelligence-beats-ai.jpg
This episode of Fast Forward was recorded in the IBM Watson Experience Center here in New York City. My guest was Rob High, the Vice President and Chief Technology Officer of IBM Watson.
High works across multiple teams within IBM, including engineering, development, and strategy. He is one of the most lucid thinkers in the space of artificial intelligence, and our conversation covered many of the way that technology is reshaping our jobs, our society and our lives. Read and watch our conversation below.
Dan Costa: What is the dominant misconception that people have about artificial intelligence?
Rob High: I think the most common problem that we’re running into with people talking about AI is they still live in the world where I think Hollywood has amplified this idea that cognitive computing, AI, is about replicating the human mind, and it’s really not. Things like the Turing test tend to reinforce that what we’re measuring is the idea of AI being able to compete with fooling people into believing that what you’re dealing with is another human being, but that’s really not been where we have found the greatest utility.
This even goes back to, if you look at almost every other tool that has ever been created, our tools tend to be most valuable when they’re amplifying us, when they’re extending our reach, when they’re increasing our strength, when they’re allowing us to do things that we can’t do by ourselves as human beings. That’s really the way that we need to be thinking about AI as well, and to the extent that we actually call it augmented intelligence, not artificial intelligence.
No compatible source was found for this media.
Let’s talk a little bit about that shift, because it’s an entirely new type of computing. It’s the evolution of computing from what we both grew up with, a programmatic computing where you would use computation to reach and answer using a very complex process, to cognitive computing, which operates a little differently. Can you explain that transition?
Probably the biggest notable difference is that it’s very probabilistic, whereas programmed computing is really about laying out all the conditional statements that define the things that you’re paying attention to and how to respond to them. It’s highly deterministic. It’s highly mathematically precise. With a classic programmed computer, you can design a piece of software. Because you know what the mathematical model is that it represents, you can test it mathematically. You can prove its correctness.
Cognitive computing is much more probabilistic. It’s largely about testing the signals of the spaces that we’re focused on, whether that is vision or speech or language, and trying to find the patterns of meaning in those signals. Even then, there’s never absolute certainty. Now, this is in part because that’s the way it’s computed, but also because that’s the nature of human experience. If you think about everything that we say or see or hear, taste or touch or smell or anything that is part of our senses, we as human beings are always attempting to evaluate what that really is, and sometimes we don’t get it right.
What’s the probability that when I heard that sequence of sounds, it really meant this word? What’s the probability that when I saw this sequence of words it meant this statement? What’s the probability that when I see this shape and an image that I’m looking at that it is that object? Even for human beings, that’s a probabilistic problem, and to that extent it’s always the way that these cognitive systems work as well.
If somebody comes to you and they have a problem that they want to solve, they think that there is a cognitive computing solution to that, they come to Watson, they say, “Look, we’re going to use Watson to try and solve this problem.” Out of the box, Watson doesn’t do very much. They need to teach it how to solve their problem. Can you talk about that onboarding process?
Actually, we should talk about two dimensions of this. One is that some time ago we realized that this thing called cognitive computing was really bigger than us, it was bigger than IBM, it was bigger than any one vendor in the industry, it was bigger than any of the one or two different solution areas that we were going to be focused on, and we had to open it up, which is when we shifted from focusing on solutions to really dealing with more of a platform of services, where each service really is individually focused on a different part of the problem space. It’s a component that, in the case of speech, is focused strictly on the problem of trying to take your speech and recognize what words you’ve expressed in that speech, or take an image and try and identify what’s in the image, or take language and attempt to understand what its meaning is, or take a conversation and participate in that.

First of all, what we’re talking about now are a set of services, each of which do something very specific, each of which are trying to deal with a different part of our human experience, and with the idea that anybody building an application, anybody that wants to solve a social or consumer or business problem can do that by taking our services, then composing that into an application. That’s point one.
Point two is the one that you started with, which is, all right, now that I’ve got the service, how do we get it to do the things we want it to do well? The technique really is one of teaching. The probabilistic nature of these systems is founded on the fact that they are based on machine learning or deep learning, and those algorithms have to be taught how to recognize the patterns that represent meaning within a set of signals, which you do by providing data, data that represents examples of that situation that you’ve had before where you’ve been able to label that as saying, “When I hear that combination of sounds, it means this word. When I see this combination of pixels, it means that object.” When I had those examples, I can now bring you to the cognitive system, to these cognitive services, and teach them how to do a better job of recognizing whatever it is that we want it to do.
I think one of the examples that illustrates this really well is in the medical space, where Watson is helping doctors make decisions and parsing large quantities of data, but then ultimately working with them on a diagnosis in partnership. Can you talk a little bit about how that training takes place and then how the solution winds up delivering better outcomes?
The work that we’ve done in oncology is a good example of where really it’s a composition of multiple different kinds of algorithms that, across the spectrum of work that needs to be performed, are used in different ways. We start with, for example, looking at the medical record, looking at your medical record and using the cognitive system to look over all the notes that the clinicians have taken over the years that they’ve been working with you and finding what we call pertinent clinical information. What is the information in those medical notes that are now relevant to the consultation that you’re about to go into? Taking that, doing population similarity analytics, trying to find the other patients, the other cohorts that have a lot of similarity to you, because that’s going to inform the doctor on how to think about different treatments and how those treatments might be appropriate for you and how you’re going to react to those treatments.
Then we go into what we call the standard of care practices, which are relatively well-defined techniques that doctors share on how they’re going to treat different patients for different kinds of diseases, recognizing that those are really designed for the average person. Then we lay on top of that what we call clinical expertise. Having been taught by the best doctors in different diseases what to look for and where the outliers are and how to reason about the different standard of care practices, which of those is most appropriate or how to take the different pathways through those different care practices and now apply them in the best way possible, but finally going in and looking at the clinical literature, all the hundreds of thousands, 600,000 articles in PubMed about the advances in science that have occurred in that field that are relevant to now making this treatment recommendation.
All those are different aspects of algorithms that we’re applying at different phases of that process, all of which have been taught by putting some of the best doctors in the world in front of these systems and having them use the system and correct the system when they see something going wrong, and having the system learn essentially through that use on how to improve its own performance. We’re using that specifically in the case of oncology to help inform doctors in the field about treatment options that they may not be familiar with, or even if they have some familiarity with it may not have had any real experience with and don’t really understand how their patients are going to respond to it and how to get the most effective response from their patients.
What that basically has done is democratized the expertise. We can take the best doctors at Memorial Sloan Kettering who had the benefit of seeing literally thousands of patients a year around the same disease from which they’ve developed this tremendous expertise, capture that in the cognitive system, bring that out to a community or regional clinic setting where those doctors may not have had as much time working with the same disease across a large number of different patients, giving them the opportunity to benefit from that expertise that’s now been captured in the cognitive system.

I think that idea of distributing that expertise, first of all, capturing it is a non-trivial task, but then once you’ve done that, being able to distribute it really across the planet, you’re going to have the expertise of the best doctors at Memorial Sloan Kettering being able to be delivered in China, in India, in small clinics, and I think that’s pretty extraordinary.
It has a tremendous social impact on our welfare, on our health, on the things that will benefit us as a society.
On the flip side, the thing that concerns people about artificial intelligence is that it’s going to replace people, it’s going to replace jobs. It’s tied into the automation movement. The thing that strikes me is, staying in the medical space, radiologists. Radiologists look at hundreds and hundreds of slides a day. Watson or an AI-based system could replicate that same type of diagnosis and image analysis. Ten years from now, do you think there are going to be more or fewer human radiologists employed in the US? What’s the impact on industries like that?
The impact is actually about helping people do a better job. It’s really about … take it in the case of the doctor. If the doctor can now make decisions that are more informed, that are based on real evidence, that are supported by the latest facts in science, that are more tailored and specific to the individual patient, it allows them to actually do their job better. For radiologists, it may allow them to see things in the image that they might otherwise miss or get overwhelmed by. It’s not about replacing them. It’s about helping them do their job better.
It does have some of the same dynamic that every tool that we’ve ever created in society. I like to say if you go back and look at the last 10,000 years of modern society since the advent of the agricultural revolution, we’ve been as a human society building tools, hammers, shovels, hydraulics, pulleys, levers, and a lot of these tools have been most durable when what they’re really doing is amplifying human beings, amplifying our strength, amplifying our thinking, amplifying our reach.
That’s really the way to think about this stuff, is that it will have its greatest utility when it is allowing us to do what we do better than we could by ourselves, when the combination of the human and the tool together are greater than either one of them would’ve been by theirselves. That’s really the way we think about it. That’s how we’re evolving the technology. That’s where the economic utility is going to be.
I completely agree, but I do think there’s going to be industries that are obviated because of the efficiency introduced by these intelligent systems.
They’re going to be transitioned. Yeah, they’re going to be transitioned. I don’t want to diminish that point by saying it this way, but I also want to be sure that we aren’t thinking about this as the elimination of jobs. This is about transforming the jobs that people perform. I’ll give you an example. A lot…